Rail predictive maintenance—one product, one user, $20million in value
Increasing iron ore production through rail track maintenance optimisation.
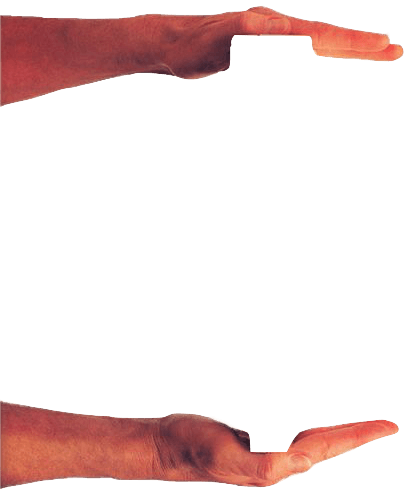
Confidential
9 months
Mining
Product Owner
Tech Lead
3 in-house developers
Snr Data Scientist
Jr Data Scientist
Scrum Master
Value Engineer
A planning tool that automatically suggests rail maintenance activities without losing the flexibility that rail engineers need to adjust schedules.
Discovery
Joining the team late
The initial team consisted of a data scientist, PO, tech lead and a developer. They were working together for ~3 months before I joined the team. In this time, an initial user interface had already been designed and development had begun.
My first challenge was to audit and re-design the interface while having minimal disruption to the team's flow and development timeline. I was working across 2 product teams with 7 developers between them, so my main priority was to keep the backlog full.
My first tasks were to…
Problem Space deep dive
Review the backlog
Conduct user testing
Audit existing UI to identify any immediate UX / UI issues
Reprioritise features that needed more design work and pull in easy wins to fill development capacity
Design
User Testing
The existing UI had not been tested yet so I quickly got in contact our key user and conducted a 45min testing session. Although this user would be the only person using this tool, I also spoke to a few other engineers and managers in his circle and conducted user testing with them.
The main things I wanted to test in these initial sessions were…
End to end user flow
Did the layout fit their mental modals?
What data was essential, what could be hidden?
Did the language used in the app make sense?
("backlog", "workgroup", "join")
UI Clean-up
Since a complete re-design wasn't an option, the user testing sessions gave me just enough information to validate certain aspects of the UI and determine what immediate changes needed to be made.
These changes included cleaning up the data, changing some of the language used, moving some features around as well as aligning to the design system and checking accessibility (colour contrast, font sizes, etc).
Figma designs before I joined the team
Figma designs after I joined the team
User flow
After the initial UI updates were made, I went back to basics. I mapped out user flows and processes to better understand where the product would sit in the rail maintenance ecosystem and to begin working on a more robust product strategy and roadmap.
High level maintenance flow & break down of focus area
Outcomes
Although this application only had one end user (Hi, Johnny!), we were able to have a $10 million impact on production.
Since every hour of production downtime equates to roughly $1million, saving just 10 hours per year had a huge impact.
Our predictive maintenance model allowed us to be more strategic with rail maintenance activities and therefore reducing possessions and shutdowns. Along with this, we were able to improve the condition of the rail which resulted in the removal of temporary speed restrictions (these are put in place when the rail condition is poor). After safety, our planning algorithm prioritised time impacts to maximise value.